The AI Boom is good for Quantum Tech
These days it is impossible to ignore the progress and growth of artificial intelligence. Like most early stage tech investors, we are often asked how this trend relates to our investment areas in physics & compute and especially about how AI relates to quantum technologies.
We see the AI Boom as a great opportunity for deep compute tech in general, and in this post we focus on its relationship to quantum technology in particular. At Quantonation, we’re seeing firsthand how these two fields drive each other forward.
The AI Compute Crunch
It’s no secret that AI has an insatiable appetite for computational power. As models grow larger and tasks become more complex, we’re seeing:
1. Huge and growing demand for compute. Training frontier models requires 1000X more compute today than it did 4 years ago.
2. Dramatically rising costs. The cost of training those models is 100X what it was 4 years ago.
3. A near-monopoly by NVIDIA, with rapid growth to a staggering market cap over $3.1 trillion.
NVIDIA Stock Price
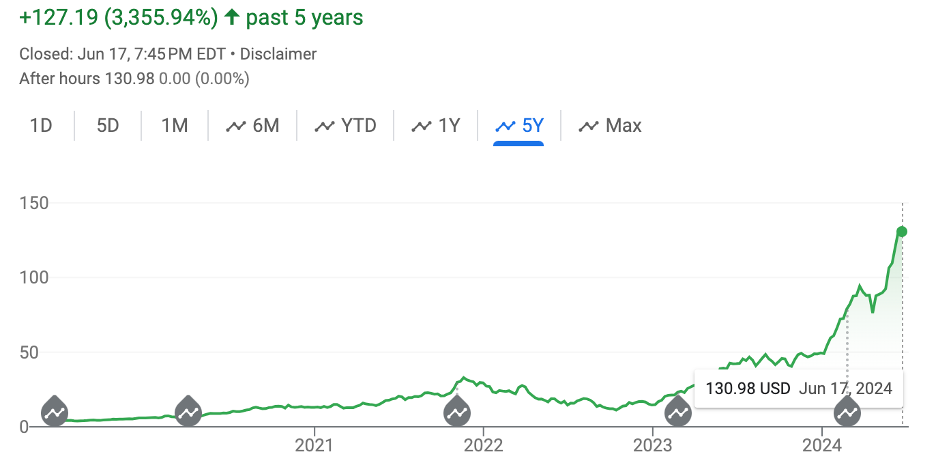
This situation creates strong incentives to explore alternatives to traditional CPUs and GPUs. There are various approaches in research and development that we are looking at for investments:
- FPGAs (e.g. Intel Agilex)
- ML ASICs (e.g. TPUs, Graphcore, Cerebrus, Tenstorrent, Etched)
- Superconducting (e.g. IMEC SPUs)
- Thermodynamic (e.g. Extropic, Normal Computing)
- Reversible (e.g. Vaire Computing)
- Optical (e.g. Lightmatter)
- Analog (e.g. Mythic)
- Biological (e.g. Cortical Labs, Catalog DNA)
- Quantum Computing
Critically, quantum computing doesn’t just offer a constant factor speedup – it unlocks entirely new algorithms and approaches to problem-solving. If you are going to unseat NVIDIA and other well funded incumbents, then you need more than just great execution. You need a fundamentally disruptive approach. Of the alternatives, quantum computing is the most disruptive, supporting new algorithms with computational complexity scalings that are not available on other hardware. You can find an overview of recently applications in this whitepaper and a list of some of these new algorithms is in the quantum algorithm zoo.
The Virtuous Cycle: AI ⇄ Quantum
Quantum technologies include more than just quantum computing. They include new technologies and products in sensing, networking and communications. Across these areas, we see places where AI and quantum technologies are mutually beneficial:
Quantum for AI
Faster training and inference. Quantum machine learning is an active research area with approaches from heuristic research on near term quantum devices to longer term research on complexity speedups for future quantum computers.
Quantum-inspired classical algorithms. Mathematical techniques like tensor networks that have been developed for quantum physics are being explored in applications for with large linear algebra in general. These run algorithms run on classical computers but make use of quantum physics techniques.
Better training data. Quantum computers could provide more accurate simulations of the physical world and quantum sensors could provide better measurements (e.g. gravimeter or magnetometry data for mining). Better data from quantum technologies can improve classical machine learning models. Further, quantum computers are good at doing machine learning on quantum data.
Enhanced privacy and security in AI computations. Quantum computers and networks support blind quantum computing, where a compute server has not access to the client’s input data, output data, or to what computation the client is asking the server to run. There are other cryptographic protocols that are being explored with some listed in the quantum protocol zoo (hosted by Quantonation portfolio company Veriqloud).
AI for Quantum
Quantum processor design optimization. Quantum processors are hard to design! Machine learning systems could help with layout and simulation.
Improved calibration and control of quantum systems. After design and fabrication, classical electronics (like those from Quantonation portfolio company QBlox) calibrate and program the quantum systems. Better machine learning can help increase the fidelity of this control, reducing errors in quantum sensors, networks, and computers.
Optimization in variational quantum algorithms. Many quantum computer algorithms are hybrid: there are outer loops where a classical computing system is doing online optimization of a subroutine that is being run on a quantum computer. Better classical machine learning could improve this.
Automated translation of classical code to quantum algorithms (think “Quantum Copilot”). For quantum computing to reach mass adoption, much classical code will eventually need to be ported over. Better AI systems for coding and transpilation could help with this.
Some Recent Examples
This symbiosis isn’t just theoretical. We are seeing examples of it across our portfolio today:
1. Qubit Pharmaceuticals is using quantum-accelerated simulations to train better ML models for drug discovery. This creates a virtuous cycle: better quantum chemistry simulations → better training data → improved ML models for pharmaceutical research.
2. Multiverse Computing has developed CompactifAI a quantum-inspired approach that significantly speeds up Large Language Model (LLM) training. This demonstrates how quantum concepts can enhance AI even on classical hardware.
3. Pasqal is leveraging analog quantum programming with their neutral atom computers to implement graph neural networks. By rearranging atoms to mimic problem structures, they’re tackling problems in logistics, social network analysis, and biological processes.
The State of Quantum Machine Learning
How can quantum computers help improve AI algorithms? While still in its early stages, quantum machine learning (QML) is growing rapidly as a research field. In 2023, there were over 4,000 publications on QML, compared to basically none 10 years earlier. This is a great start, but we are still very early on the scale of other research areas. In 2023, QML is only about 0.67% of all published machine learning papers.
These numbers speak to growing interest in the field but should give us the perspective that things are still very early and there is a lot more to figure out.
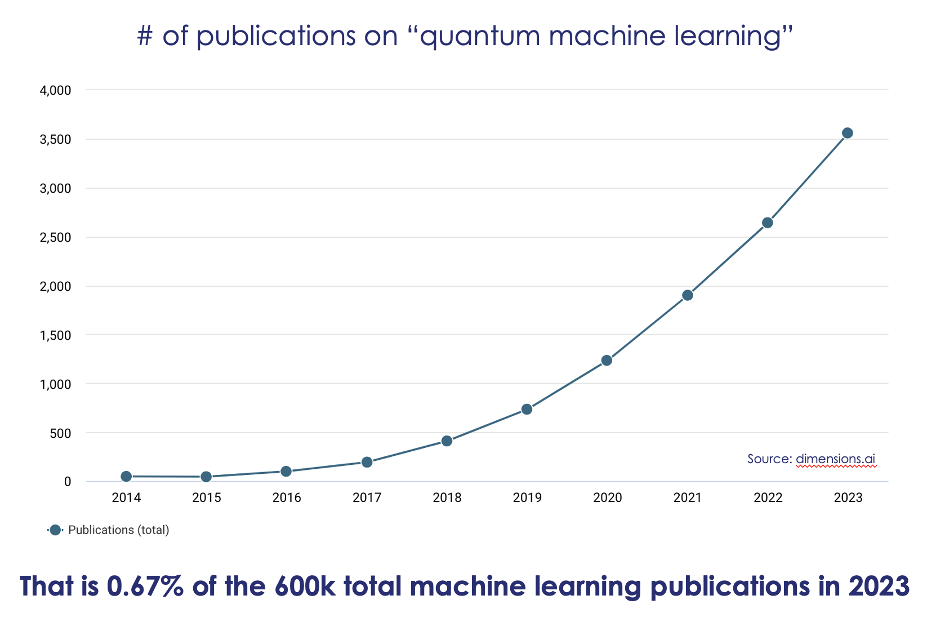
Theoretical quantum speedups in ML are promising, but there are practical challenges:
– Many approaches require significant quantum memory
– Some examples are esoteric and hard to generalize
– It’s difficult to compare performance at scale
Classical ML benefits massively from empirical results: programming ideas and trying them against benchmarks on larger and larger systems. Indeed, a major research theme in modern machine learning is that “scale is all you need”, i.e. just more and bigger computers and not fundamentally new algorithms.
This presents a difficulty for quantum machine learning research where almost all our quantum computers so far have been subscale, i.e. small enough (<50 qubits) that they could just be simulated by classical computers and so can’t do fundamentally new computations.
Over the next few years, we will see more and more deployments of quantum computers that are not trivially simulable. These will be powerful enough to support interesting empirical tests of QML algorithms in practice. Only then will the real race in QML application research have started.
Looking Ahead
We anticipate that the current AI boom will drive increased interest in quantum technologies. Quantum-inspired classical algorithms are already making an impact, and we expect the future generation of quantum computers to usher in practical, novel quantum machine learning techniques. Still, to get to realize the potential over the long term will require focused, sustained investment and hard work.
At Quantonation, we’re excited to support the founders and teams at the forefront of this convergence of AI and quantum tech, backing the startups and technologies that will shape the future of computing.
This blog post is based on internal Quantonation research and publicly available information. For the latest developments in quantum technologies and AI, visit [www.quantonation.com] or follow us on social media [X] [LinkedIn].
About Quantonation
Quantonation is an early stage venture capital fund investing globally in novel technologies based on advances in physics and/or computing. With +€150m of Assets Under Management and an international team of both scientists and investors Quantonation aims at supporting the transition from lab to commercial products. Quantonation is headquartered in Paris, France, and in Boston, USA, with investments in Europe, North America and Asia-Pacific.